CRAPOLA design principles for quantitative data
Robin Williams (from her website: “writer. teacher. mom. not the actor”) has developed the widely cited CRAP design principles: Contrast, Repetition, Alignment, and Proximity. These are powerful general purpose design principles, but a few additional principles are helpful with respect to the display of quantitative information.
Inspired by the work of Edward Tufte, I propose three additional rules be added to complete CRAP: Obviousness, Lightness, and Accuracy. The resulting CRAPOLA design principles for quantitative data are:
Contrast: avoid similar elements (type, color, size, shape, etc.); if they’re not the same, make them very different.
Repetition: repeat visual elements throughout to organize and unify.
Alignment: every element should have some visual connection with another element.
Proximity: group related items close together to facilitate comparison.
Obviousness (clarity): clearly communicate the data.
Lightness (efficiency): show the data and nothing else.
Accuracy (precision): do not omit or distort data.
Reporting versus analytics
“We are drowning in information but starved for knowledge.”
- John Naisbitt
For the love of statistics, please stop using the words “reporting” and “analytics” interchangeably. Reporting is the display of information. Analytics is the interpretation of information. Analytics is the process that turns information into insight, reporting into understanding.
Every time someone uses the word “analytics” to mean “reporting,” a statistician loses its wings. If you ever need evidence that the misuse of these terms is widespread, just think about how many statisticians you’ve met who have wings. I’ve never met one. All the wings are gone.
Here are a few of my favorite illustrations of the difference between reporting and analytics:
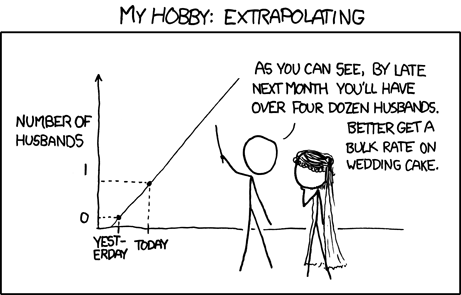
Source: xkcd.com
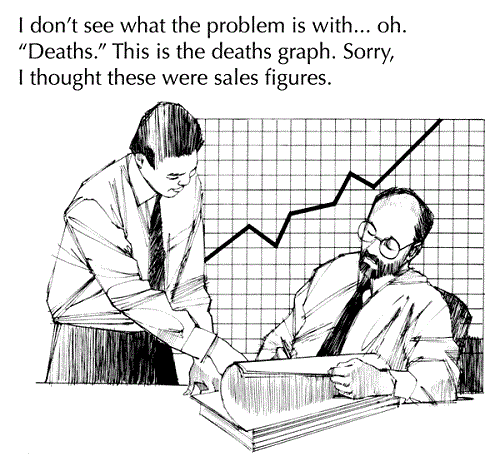
Source: marriedtothesea.com
Since analytics solves problems, which is a lot sexier than reporting’s goal of presenting information, companies with reporting solutions have a vested interest in conflating the terms. Phrases like “analytics solution,” “analytic database,” and “advanced analytics” are used with the intent to confuse, to convince users to invest in technology over thought. A typical exchange goes as follows:
Reporting tool salesperson: “I have an analytic database.”
Business person: “Thank God! All my problems are solved.”
Here are a few of my favorite examples of oversells in the reporting world:
And don’t get me started on the phrase “business intelligence,” which has been used so many different ways with so many different connotations that it has been rendered virtually meaningless. If you ask ten people in the BI industry what “business intelligence” means, you’ll get twenty opinions. In case you’re looking for something to do this weekend.
Uber’s newest data visualization is uber misleading
The description of surge pricing on Uber’s website begins with the carefully crafted sentence: “Uber rates increase to ensure reliability when demand cannot be met by the number of drivers on the road.” What that really means is that fares are higher at the worst possible time.
If it weren’t for surge pricing, Uber would be America’s most loved corporate darling. Okay, that’s not true at all. What is true is that Uber’s practice of surge pricing is widely disliked. This past New Year’s Eve surge ushered in some particularly bad press (e.g. this and this), so Uber’s on a mission to get back its users’ love.
I woke up this morning to an e-mail from Uber justifying and explaining the practice:
Notice anything funny about the graphic toward the bottom of the e-mail? If not, take a moment to look at it again.
See it? There’s a huge mismatch between the prices displayed and the widths of the corresponding bars.
Here are the prices of the illustrative rides and bar widths in Uber’s e-mail (as displayed in Gmail in the latest version of Google Chrome). Also included for each value is percentage change compared to an uberX ride with no surge.
The graphic contains three clear visual lies, each of which skews perception in Uber’s favor:
- The uberX ride with 1.5x surge is 41% more expensive than the uberX ride with no surge, but the corresponding bar is only 13% wider. The effect is that surge pricing is portrayed as less of a jump than it actually is.
- The uberX ride with 2.0x surge is 83% more expensive than the uberX ride with no surge, but the corresponding bar is only 47% wider. The effect is the same.
- The uberPOOL (Uber’s ride-sharing service) ride is 47% less expensive than the uberX ride, but the corresponding bar is 58% narrower. While uberPOOL is significantly cheaper than uberX, the effect portrays uberPOOL as an even better deal than it is. (It is no secret that Uber wantsmore riders to pool.)
I repeat: Uber’s poor visualization makes it look like surge pricing isn’t as much of a jump as it actually is, and that uberPOOL is a better deal than it actually is. That is not good.
Uber’s bar chart doesn’t just use tricks of the eye as many misleading visualizations do. The widths of the bars purporting to represent price simply don’t correspond to the prices in any way. From a data visualization perspective, this is a clear misrepresentation of the facts. Regardless of whether this misrepresentation was intentional or an oversight (to be clear, this article takes no position on this issue), this will almost definitely have an impact on consumer behavior.
Instead of graphing as an afterthought, recognize that visualizations can have real consequences. Which, if you are seeking to spread the truth, is a challenge worth some serious consideration. And which, if you happen to be a propagandist, is pretty darn convenient.
How to hire data scientists – free white paper
Check out Bitten Labs’ new white paper on how to repeatably identify top data science talent. The process has been informed by hiring processes at many organizations with effective data science groups, refined through several iterations, and tested with hundreds of applicants. The white paper is a significantly expanded version of our popular blog post.
The white paper can be accessed here: How to Hire Data Scientists.
Happy hiring!
Hiring data scientists: the data problem
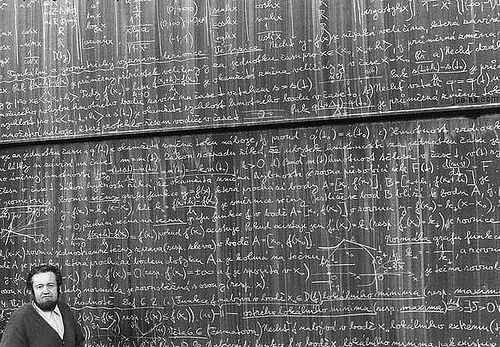
Image courtesy of misterbisson
Data scientists: many companies want them, but few know how to identify them. A widely-cited 2011 McKinsey report highlights the steep shortage of analytical talent capable of extracting value from data. With demand outpacing supply and companies hiring faster than they can find good people, the data scientist title is rapidly becoming diluted as it spreads. As a result, the title’s proliferation in resume experience sections over the past few years isn’t making it any easier to find qualified candidates.
I’ll be sharing some tricks of the trade that can help identify the elusive data scientist, a mythical beast with the power to turn data into not just insight but dollar signs. The current post will focus on what I’ve found to be a key component to interviewing data scientists: the data problem. Many prospective data scientists can talk before they can walk so, unless you are otherwise certain of their skills, requiring job candidates to prove their mettle is a must.
The process we’ve developed at Litle, where I founded and continue to run the data science group, has been refined through several iterations and proven invaluable with dozens of job applicants over the past few years. The basic framework is simple: once candidates have passed an initial screening, we provide some data and a problem statement and ask them to prepare slides and present the results to a diverse group of interviewers.
The rest of this post will delve deeper into (1) finding a good data problem, (2) engaging candidates while they’re working on the problem, (3) hosting the presentation, and (4) evaluating the candidate.
Phase 1: Finding a problem
At Litle, we give prospective data scientists a table containing around a million rows and ten columns of data directly (modulo anonymization) from our database. This dataset is accompanied by a dense and polished one page problem description – divided roughly equally into background information, data definitions, and problem statement – that describes a research problem to which we’ve dedicated many scientist-months and from whose results we’ve built a successful analytics-based product.
Designing a good problem is critical to success. The particulars will (and should) vary, but here are some general pointers:
Make it relevant. Gear the problem statement to identify skills relevant to your business; there is no one size fits all data problem. Ideally, use a real dataset and pose a problem with which the interviewers are highly familiar, to facilitate conversation and evaluation.
Make it fuzzy. Refrain from providing clear success metrics. Well-defined problems are rare in the real world and not typically the purview of the data scientist. To mirror what makes a data scientist successful on the job, identifying the problem – discovering the right questions to ask of the data given limited background knowledge – and defining success metrics for a solution should be part of the challenge. However, make sure to keep definitions clear so you’re all on the same page. In addition to asking for a solution to the problem at hand, explicitly encourage the candidate to present other insights found in the data; a good candidate won’t let this distract from the main point.
Make it hard. This goes hand in hand with “make it relevant” and “make it fuzzy”: you probably aren’t looking for a data scientist to solve easy, well-defined problems for your business. Make sure a substantial amount of preprocessing is necessary before a standard model is applied.
Ask for action. Require actionable insights from the data. Someone who finds cool things in a data set all day long may be a great help in generating marketing content, but recommending actions is what will help your business make money and ship product.
Use complex, real world data. Assuming the hire will be working with real world (as opposed to simulated) data, which is usually the case, make sure the data has some messiness. Missing values are a must (don’t try to make them go missing yourself, as this process will invariably leave undesirable artifacts behind). Unlabeled factors (e.g. “Factor A,”, Factor B,” etc.) are nice, as candidates won’t have the easy out of relying on logic alone to dictate models and better candidates will attempt to discern the meanings of these factors and of individual values.
Phase 2: Guiding the solution
There are many opportunities during the work phase to have a big impact on the outcome.
Set expectations. Be open and honest from the start on your expectations around desired output, project timeline, interactions, and audience. Aim for a quick turnaround from beginning to end; one to two weeks usually works well. Most candidates who make it all the way to the presentation claim they spent two to three full days between doing research and preparing slides.
Communicate early and often. Encourage candidates to send questions, plans for vetting, and even presentation drafts. These interactions make the process go more smoothly and allow early appearance of green and red flags. We’ve learned from work plans that certain candidates are exceptionally organized. We’ve also learned from early presentation drafts that others are not going to work out. In return, provide plentiful feedback and advice, as you (presumably) would on a new employee’s first project.
Start evaluating early. Evaluation starts the moment you introduce the notion of a data problem to a candidate. A great deal of useful information can be gathered before the candidate even shows up for the presentation.
Pull the plug early if it won’t work out. Be clear in advance that the invitation to present is conditional on strong evidence of potential success. Don’t invite anyone to give a presentation until you’ve seen this evidence. If there is reasonable evidence to the contrary then thank them for their time and pull the plug. Time is precious.
Be nice. Be polite and appreciative as candidates will be putting a lot of their own time into something that may very well not result in a job offer. Also, remember that recruiting is a great way to build your network.
Leave the tools up to the candidate. A good data scientist should be resourceful enough to find freely available tools to work on the problem, and most should already have some favorites on hand. You don’t need to, and shouldn’t, provide software. Advice is of course fine.
Phase 3: Hosting the presentation
An interactive presentation is key to successful evaluation.
Keep it short and follow up with breakouts. An hour including questions works well. Starting with a presentation to all the day’s interviewers has the added bonus of making subsequent meetings (one on one or in small groups) more efficient.
Have the right people in the room. At least one or two strong data scientists absolutely need to be present. If you don’t have any data scientists in house, make sure to bring in a trusted consultant (who should also craft the problem statement). This isn’t something you should try without the proper expertise. Beyond this requirement, tailor the audience to the hire’s role. A mix of data scientists, product owners, engineers, and solutions experts may work well for a product research and development role, for example.
Ask the easy questions. Get a sense for whether the candidate has an overall understanding of the data and the business problem at hand. A good data scientist will have a basic sense for the data after spending several hours with it, and practically minded candidates will have given the business some thought.
Ask the hard questions. Don’t be afraid to ask a question that’s too difficult to answer completely. Soliciting some speculation is a good thing. The good candidates will be able to say something meaningful and, just as importantly, will understand and state their limits.
Make it interactive. Challenge what the candidate is saying. Ask for an alternate explanation when something isn’t perfectly clear. If a machine learning method is applied, ask the candidate to explain it in terms everyone in the room can understand. Data scientists worth their weight generally excel at common sense explanations. A quick round of introductions can help break the ice and allow the presenter to better contextualize questions.
Add information. Provide information during the presentation that the candidate didn’t have before. A strong applicant should be able to roll with the punches, incorporating and synthesizing new information on the spot. Changing information and assumptions are part of the job.
Ask for next steps. Science is never done. What would the candidate do if given more time?
Evaluation
While evaluation should start before the presentation, this section will focus on the presentation. Look for:
Big picture thinking. The candidate should spend most of the presentation on understanding the data and problem: visualization, data cleaning, asking fundamental questions to assess bias, introducing frameworks, diagramming, discussing assumptions, etc. Back of the envelope calculations are a good sign. Better machine learning people will usually spend the bulk of the time talking about feature selection and cover the modeling in a few minutes. Clarity on the big picture should be evident, and your own understanding of the data should improve from attending. Simplicity is king.
Storytelling. Distilling a complex dataset into a simple and meaningful story is a key skill for data scientists. As an apocryphal quote often attributed to Einstein goes, “Everything should be made as simple as possible, but not simpler.” Approximation is one simple example of this: a candidate should verbalize numbers to the precision at which they best support the story without distraction (“twelve million” or “around ten million” instead of “eleven million, seven hundred fifty four thousand, eight hundred twenty one”).
Communication. Since subtle communication is common for data scientist roles, expect strong communication skills. Superb scientific communication is important for every hire, and excellent business communication is key for those interacting outside the data science group. Even if some interviewers don’t get all the technical intricacies of the presentation, everyone should get something significant out of it. The slides should be well structured and look passably decent, with a significantly higher bar for business-facing roles. The candidate should display openness to new ideas and be able to address constructive feedback without defensiveness. Humility is critical to success in the data scientist role.
Productivity. Look for evidence of productivity while still demanding simplicity in communication of results. Prepare to be amazed here; some stronger candidates do a pretty incredible amount of work in a short time. Depending on the tool used, high productivity (taken together with logical reasoning, organization, a clear framework and consistent use of terminology, etc.) is one clue that the candidate is or has potential to become a strong coder.
Creativity. Expect to learn something new (and non-canonical) from the presentation. The candidate should look at things at least a little bit differently than anyone else in your organization and go beyond textbook methods.
Connoisseurship of good science. You’re hiring a scientist, so evaluate the candidate on rationality and skepticism. The candidate should pay explicit attention to issues of experimental design and validation, causality, bias, normalization, significance, etc. If a model is created the data should be split into modeling and testing sets, time trends should be interrogated to make sure results are meaningful, and so forth. Talking in terms of black boxes and magic is not a good sign. Measured confidence is a positive trait but humility in the face of reason is equally as important for a data scientist role.
Curiosity, passion, and resourcefulness. These are traits that help employees grow; if you find a high potential individual who possesses them in spades but is missing some experience then the hire may be well worth the training effort. Candidates with these skills will be able to figure out more about the data set, the problem, and your business than you expected given the information provided. The Internet and scientific literature are their friends. Pay attention to candidates who say they enjoyed the exercise, ask for ways to improve their solution, or express interest in continuing the investigation.
Practicality. The candidate should define success metrics that make sense. Conclusions should be actionable: they should solve meaningful business problems. The candidate should possess the mentality to get initial actionable results quickly and iterate, particularly if you are hiring for a product development role.
Attention to detail. The work should be thorough and the presentation should be relatively free from logical and typographical errors. The candidate should notice irregularities in the data and detect and correct for systemic biases. For example, in the case of time series the candidate should account for series cut off by the end of the sample period. Pay particular attention to how the candidate handles missing data.
Performance expected from a new employee. What you see is what you get. The presentation is a very good indication of what the output of a candidate’s first few projects will look like. You can blame performance less than what you’d expect from a new employee on any number of factors, but in practice job applicants put their best foot forward. Make sure the output works for you before proceeding.
Wow factor. Finally, expect to be wowed. Taken together, these criteria may seem like a steep order, but keep your expectations sky high. Data scientists who can make a real impact on your business are not junior employees and, to succeed, must excel in many of these areas. Despite the talent shortage, there are still good people on the market. If you have rich data sets and impactful problems, you should be able to attract talent. Just be ready to pay for it.