Seven ways to avoid the seven month data science itch
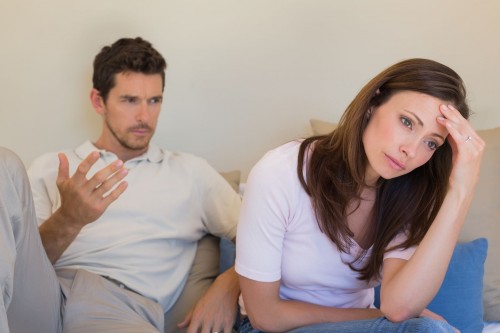
Image courtesy of Relationships Unscripted
It is a story as old as data science: company has valuable data asset, company hires data scientist, data scientist finds insights, company is thrilled, company can’t figure out what to do next. The months after a company’s data first goes under the microscope can be an exciting time, but as initial goodwill fades the thrill of young love can turn into a seven month itch.
The itch often starts as a mere tickle, but indicates very serious underlying issues. If you have heard the question “how can we use data science here?” around the office more than once, it is likely that your organization is about to enter the trough of disillusionment. Seemingly small problems have a way of revealing themselves to be much larger underneath; the seven month itch can be deadly to a company’s data science efforts.
The seven month itch is felt by both the data science team and its collaborators. The result is a lack of effectiveness, though the perceived root causes differ across the aisle.
Data scientists typically see the seven month itch as a lack of business prioritization and engineering resources to bring their vision to fruition. They often have a seemingly endless stream of ideas and are able to find promising insights in the data, but see the business as unsupportive. This is probably the most common issue expressed by young data science teams.
Collaborators of the data science team, on the other hand, typically see the seven month itch as a lack of practicality and business savvy on the part of the data scientists. Data scientists are frequently derided for being too academic, for privileging theoretical interest over practical relevance. This is often the case.
Both data scientists and their collaborators feel they are not getting what they need from the other party.
For product-facing data science teams, for example, product design is a common area where each side feels the other should have most of the answers. Data scientists assume product managers should be able to spearhead the translations of their predictive models into functioning systems, and product managers assume data scientists should guide the product realization process. In reality, building data products is best done as a team effort that incorporates a variety of skillsets (see, for example, DJ Patil’s Data Jujitsu: The Art of Turning Data into Product).
Communication disconnects – the primary source of the seven month itch – are widespread and detrimental. Generally scrappy folks, data scientists often find ways to scratch the itch. But treating the underlying cause is the only effective long term solution.
As a start, we propose seven things that data science teams and their managers can do to avoid the itch altogether. While these suggestions are targeted toward fairly young teams that are product facing, they should be generally applicable to more experienced and decision support oriented teams as well. These recommendations can help align the business and data science stakeholders, justify dedicating company-wide resources to data science projects, and generally innovate more effectively.
#1: Tie efforts to revenue
Tie data science efforts directly to revenue. Find ways to impact client acquisition, retention, upselling, or cross-selling, or figure out ways to reduce costs. There are invariably countless ways to do this, and the method makes less difference than the critical pivot of allowing data science to be a revenue generator rather than a cost center. Executive sponsorship is critical for the success of data science efforts, especially due to their interdisciplinary and collaborative nature. Some organizations may highly value making progress toward other critical business goals, and making a dent in such goals can be a perfectly valid substitute, but focusing on projects that will impact the company’s bottom line is the surest universal way to get the attention of senior management.
#2: Generate revenue without help
Generate revenue streams prior to requesting any engineering effort. Charging for one time or periodic reports or analyses delivered via Excel or PowerPoint, for example, can be useful in getting attention and buy-in from the rest of the organization.
#3: Strengthen the core
Focus first on improving the organization’s core products, the products that generate most of the revenue. The numbers are bigger there, so having a bigger revenue impact is easier.
#4: Strengthen client relationships
Generate a more consultative relationship with clients through analytics, which will make data science indispensable to account managers and have the potential for many revenue related benefits. For example, do one off analyses for clients to help them improve their use of the product or alleviate other pain points, and track progress closely. Sometimes these partnerships will generate big wins for clients, and sometimes they will be generalizable. As a result, they can be an excellent source of battle-tested business cases, which stand a better chance of getting funded.
#5: Kickstart big projects with quick wins
Don’t forget about the low hanging fruit. Data scientists are often tempted by the hard problems, but quick wins can help justify a longer leash. Instead of going straight to creating robust decision recommendations for clients, for example, surface some basic data visibility to support their decisions, or give them A/B testing capability if applicable. This first step may not be interesting work from a data science perspective, but is can establish a new client need (e.g. “I don’t know what to do with this information or even what to A/B test; help me optimize this decision”) that data science can then step in and solve. An iterative approach works very well for developing data (and many other types of) products.
#6: Measure customer value
View your products in the light of customer value. You can often justify high prices for data products because they add demonstrable value for clients. Customer value can be data science’s best friend; giving the business a baseline level of visibility into customer value can open new worlds, and should probably be an even higher priority than building any specific data product. Create customer value metrics for the business, separately tracking core products and products that add measurable value. Ideally, give an executive responsibility for goals based on these metrics.
#7: Measure innovation
Create innovation metrics for the business, such as the percentage of revenue from products that are at most one year old, or the percentage of revenue from products that draw their value from analytics. Ideally, give an executive responsibility for goals based on these metrics.
Many organizations have most of the elements necessary to benefit greatly from data science. These recommendations should help provide a common language between the data science team and its collaborators, which is all that is necessary to avoid the seven month itch. No scratching needed.
comments
Leave a Reply